About the Author :
Monisha Eadala is an India Policy Advisor at WisdomCircle. She is a public policy analyst with expertise in international development and environmental management. With a background as a former World Bank consultant and recent experience at a clean tech startup, she is deeply passionate about driving global change through evidence-based policy solutions. Monisha brings a unique perspective, having worked across four distinct sectors—business, government, non-profits, and international organizations—around the world. She holds a Master’s in International Development Policy from Duke University.
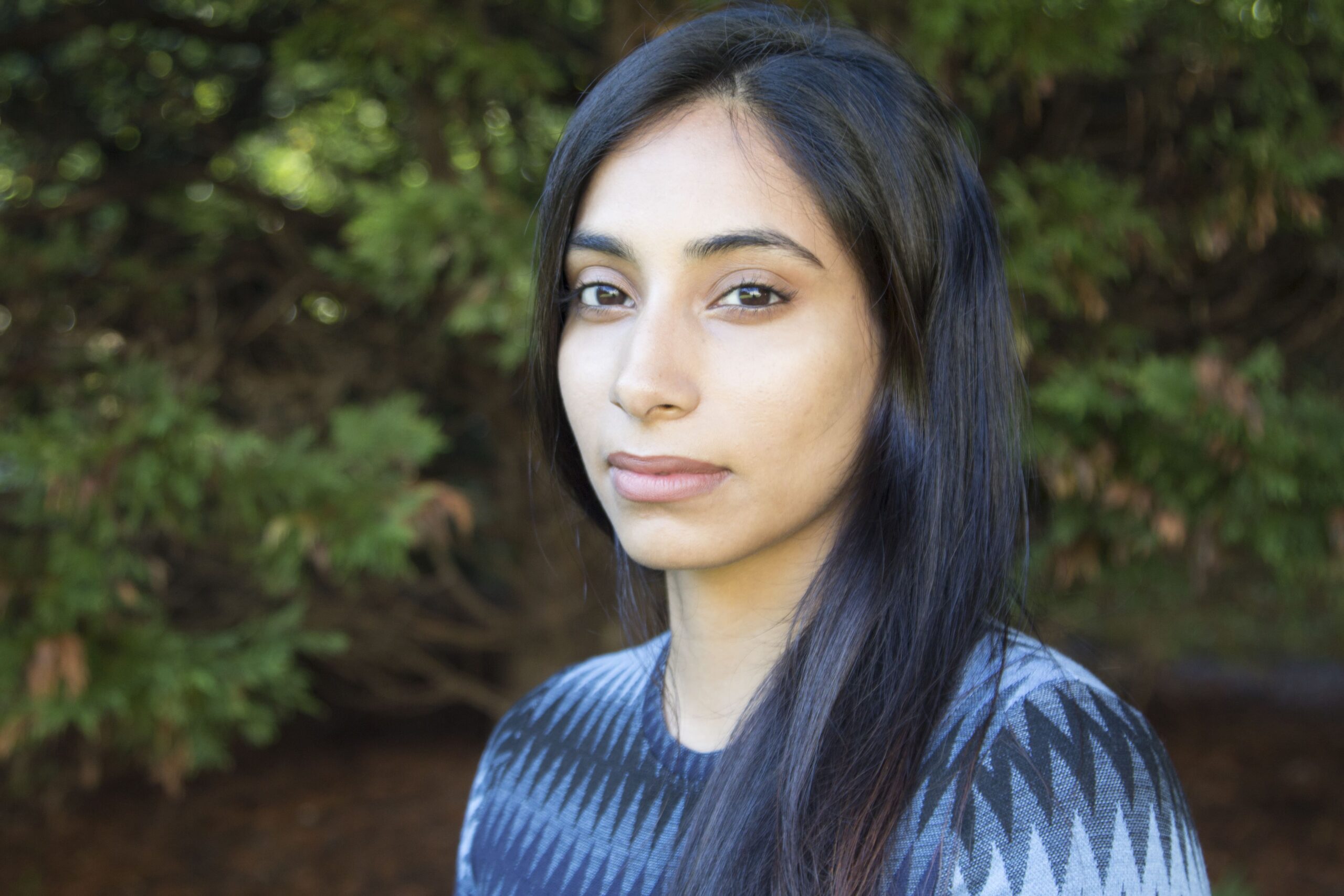
Artificial Intelligence (AI) is transforming every industry—from education and healthcare to finance and urban planning. But alongside its promise come serious challenges: algorithmic bias, lack of explainability, accuracy concerns, privacy risks, inefficiencies, limited practicality, adverse environmental impact, and regulatory uncertainty.
To meet these challenges, AI needs more than just cutting-edge code. It needs wisdom. And one of the most overlooked sources of it is retired professionals, also known as the wisdom generation (WisGen).
With decades of experience across fields like law, medicine, education, engineering, public policy, and ethics, WisGen bring essential real-world judgment, contextual understanding, and deep domain expertise. Their insight is exactly what’s needed to shape AI that is not only powerful, but responsible, human-centred, and trusted.
1. Bias Isn’t Just a Bug—It’s a Mirror
AI systems often inherit the biases in their training data, reinforcing existing inequalities—from hiring algorithms that disadvantage certain groups to facial recognition tools that misidentify minorities (Times of India, 2024; Mariwala & Sundharam, 2025; AI Multiple, 2025).
WisGen can play a vital role in addressing AI flaws at both the design and data levels. Who better to identify and correct systemic biases than those who have experienced—and in some cases shaped—the systems where the biases originated? A deeper, lived understanding of bias is essential to tackle it at the root in the algorithms we build.
Organizations like the World Economic Forum and IEEE advocate for diverse, non-technical voices in AI governance—precisely the role WisGen can fulfil (WEF, 2023; IEEE, 2019). For instance, imagine a retired civil rights lawyer reviewing a law enforcement AI tool, or a former HR director identifying demographic gaps in recruitment data. Their contributions help root out inequity before it’s embedded in the system.
WisGen can contribute by spotting underrepresentation in training datasets, serving on ethical review boards, mentoring developers on fairness and systemic inequality and designing inclusive algorithms from the start.
Bias in AI isn’t just a technical flaw—it’s a social issue. Retirees offer lived experience in managing systemic inequality, making them essential in creating fair, just and inclusive algorithms.
2. From Black Boxes to Real Answers
AI’s lack of explainability—especially in healthcare, law, and finance— creates critical challenges for communication and real-world usability. Deep learning models may predict outcomes, but can’t always explain “why” they did (Hinchliffe, 2023; Bain, 2024).
Retired compliance officers, lawyers, and auditors understand the importance of clear documentation, proper justification, and transparency. Their skills can help transform opaque AI outputs into clear, auditable decision logs that not only meet legal standards but also build AI’s credibility.
Communication is just as critical as compliance. Consider a retired nurse evaluating an AI diagnostic tool who says, “This explanation won’t work in a real ER.” That insight is invaluable. These professionals can act as translators between complex technical systems and the real-world environments they serve—ensuring explanations resonate with frontline users, not just engineers.
By bridging the gap between algorithmic decision-making and human understanding, retirees help make AI not only smarter but more accountable, accessible, and easy to understand.
3. Guardrails to Prevent AI Hallucinations
Yes, AI can hallucinate, and it absolutely needs boundaries to be set—especially in high-stakes or sensitive contexts. In large language models, “hallucination” refers to the confident generation of false, misleading, or entirely fabricated information. An AI might invent a quote, provide inaccurate statistics, or fabricate non-existent legal or medical advice. This erodes user trust—particularly in fields where precision is non-negotiable.
Two notable cases underscore the concern:
- In law, the case of Mata v. Avianca revealed that ChatGPT had fabricated legal citations and quotes, leading to flawed legal research and disciplinary action (Stack, 2023).
- In science, Google’s chatbot Bard falsely claimed that the James Webb Space Telescope captured the first image of an exoplanet—a claim directly contradicted by NASA’s records (Vincent, 2023).
These aren’t cases of intentional deception—they’re side effects of how language models function. AI doesn’t “know” facts; it predicts language based on patterns in its training data. When that data is inconsistent or a prompt is vague, hallucinations are more likely. To mitigate these risks, experts emphasize the need for clear boundaries—such as explicit instructions, rigorous validation mechanisms, safety filters, and tight domain constraints. Here, the WisGen may be uniquely qualified to help.
With decades of experience making fact-sensitive decisions in various fields, they are ideally positioned to:
- Improve data integrity by reviewing training sets to ensure the inclusion of accurate, credible, and diverse sources.
- Validate models through output testing, spotting hallucinations based on professional expertise and contextual accuracy.
- Offer ethical oversight, helping developers understand the consequences of false information in real-world scenarios.
By leveraging their knowledge, the AI industry can ultimately build more trustworthy systems.
4. Privacy in a World of Leaks
AI systems feed on massive volumes of data—much of it personal, proprietary, or sensitive. While this data fuels more powerful and accurate models, it also opens the door to serious privacy risks.
In recent years, several incidents have spotlighted these vulnerabilities:
- In 2020, OpenAI’s GPT-2 was found to reproduce private data, such as emails and phone numbers, embedded in its training set (Bender et al., 2021).
- A 2023 study showed that models trained on public code repositories like GitHub could inadvertently expose API keys and passwords left in commits—raising critical concerns about AI’s security hygiene (Toulas, 2024).
These lapses aren’t just technical glitches—they’re signals of broader systemic gaps in privacy governance, data vetting, and risk management. That’s where legal, compliance, and cybersecurity WisGen bring immense value. Having witnessed and managed real-world data breaches, they understand the legal, operational, and reputational consequences of weak data protections.
WisGen can support privacy-conscious AI development by:
- Auditing training data pipelines, ensuring that sensitive personal data is properly anonymized or excluded before ingestion.
- Designing risk mitigation protocols, informed by established regulatory frameworks like HIPAA, GDPR, and SOX.
- Advising on ethical data use, including user consent, transparency policies, and responsible data stewardship.
- Serving on AI ethics and privacy review boards, bringing real-world, cross-disciplinary judgment to oversight discussions.
- Embedding privacy-by-design principles into development teams through hands-on training and policy mentorship.
Their institutional memory—of what’s gone wrong and how it was fixed—is an untapped asset in building AI systems that respect user privacy, comply with the law, and build long-term security.
5. Mentorship: Closing the Skills Gap and Promoting Efficient Knowledge Transfer
As AI continues to evolve, the demand for expertise in ethical design, interdisciplinary thinking, and regulatory frameworks is growing faster than the talent pool can keep up (Early, 2024; Marr, 2018). In response, retired engineers, actuaries, and project managers are stepping up as mentors, leveraging their real-world experience to help younger AI professionals avoid common pitfalls and build responsible, scalable systems. Programs like MIT’s Mentor Network and MentorCruise are already connecting retirees with rising AI talent to bridge this gap (MIT Venture Mentoring Service, n.d.; MentorCruise, n.d.).
Another powerful example of how AI can support knowledge transfer is McKinsey’s internal tool, Lilli (Evolving AI, 2023). By synthesizing a century’s worth of historical documents and expert interviews, Lilli enables consultants to access critical insights, identify experts, and generate concise, actionable summaries—allowing them to focus on high-value, strategic work. This approach highlights how AI can complement mentorship by facilitating the efficient transfer of knowledge.
Companies looking to adopt a similar model can:
- Begin digitizing and curating their institutional knowledge by interviewing their previous and current senior employees and documenting their experiences.
- Then start pairing AI tools with mentorship programs to enhance the learning process. For instance, WisGen could collaborate with AI to offer personalized guidance, ensuring that valuable expertise is passed down effectively.
By combining AI-driven data synthesis with the mentorship of experienced professionals, organizations can cultivate a more agile, skilled workforce, all while preserving the wisdom and insights of their senior talent.
6. Human Context Is Important
AI doesn’t understand nuance—it learns patterns. But in fields like mental health, law, and education, nuance is everything (Singla et al., 2024). An AI might recognize correlations, but it cannot grasp intent, emotion, or the social consequences of its outputs. The absence of human sensibilities in these domains can lead to emotionally tone-deaf, overly rigid, or even harmful outcomes—diminishing relatability and effectiveness.
This is where the WisGen can bring irreplaceable value: real-world judgment, domain-specific empathy, and deep contextual insight gained through decades of frontline experience.
A retired social worker can help build trauma-sensitive AI for counselling. A former teacher might guide an AI tutoring platform to adapt to different learning styles and emotional cues. A retired judge could flag overly rigid logic in a sentencing algorithm that fails to account for mitigating life circumstances.
WisGen can help bridge the gap in AI’s understanding of human complexity by:
- Contributing to design reviews that identify emotionally or ethically insensitive outputs in high-impact sectors like healthcare, education, and social welfare.
- Advising on edge cases that demand human discretion, such as interpreting trauma responses, understanding cultural contexts, or addressing developmental differences.
- Guiding AI to navigate complex social realities, including issues related to race, age, disability, and systemic power differences.
- Conducting scenario testing that simulates high-empathy environments—like classrooms, courtrooms, or counselling sessions—to assess AI behaviour in these delicate settings.
- Training AI teams on emotional intelligence and social nuance, embedding wisdom drawn from lived experience to help systems act not only with accuracy but with humanity.
By embedding lived human complexity into the development process, retirees help ensure AI behaves less like a calculator—and more like a considerate companion. Their contributions are not just helpful—they’re essential in building systems that truly serve people with compassion and credibility.
7. Testing AI in the Real World
What works in the lab can fail miserably in the real world (Stanford Institute for Human-Centered Artificial Intelligence, 2024). AI models often perform well under ideal, controlled conditions, but break down in unpredictable, high-stakes environments.
This is where WisGen can bring unmatched value: they’ve spent careers making judgment calls in complex, high-pressure scenarios where consequences are real and stakes are human. Unlike theoretical testers or data scientists working behind screens, these professionals know how things actually unfold—in emergency rooms, air traffic control towers, logistics hubs, and courtrooms. Their knowledge of operational constraints, edge cases, and human variables can make or break the success of an AI system’s deployment.
For example, a retired doctor may flag how an AI diagnostic tool overlooks symptoms in minority populations. A former air traffic controller might notice how a predictive system underestimates human communication delays. A retired supply chain executive could raise concerns about algorithmic decisions that ignore union-mandated rest periods—putting both safety and legal compliance at risk.
WisGen can support AI readiness by:
- Running field-realistic stress tests to identify where models may fail under pressure, uncertainty, or time constraints.
- Evaluating user interfaces for clarity and usability, particularly for frontline workers under stress (e.g., ER nurses, dispatchers, pilots).
- Identifying critical edge cases often missed in training data—such as medical conditions more common in underrepresented populations or legal exceptions rarely codified in training datasets.
- Spotting operational blind spots, like how an AI tool may violate local agreements, aviation safety codes, or ethical standards of practice.
- Participating in real-world pilots and feedback loops, translating model behaviour into frontline implications before full-scale rollout.
Their feedback isn’t hypothetical—it’s reality-checked. By involving WisGen in AI testing and deployment, organizations can bridge the dangerous gap between theory and reality, ensuring that systems don’t just work—but work where it counts.
Other Areas
Beyond the core challenges of development and deployment, the WisGen offers significant value in areas critical to AI’s broader impact. In sustainability, retirees from manufacturing, logistics, and systems engineering bring decades of experience in lean design, operational efficiency, and scalable systems. They are often trained to do more with less. Their insights can help reduce AI’s environmental footprint—through smarter compute usage, greener data centre infrastructure, and optimized hardware logistics (Van Zyl, 2024).
In policy-making, retired judges, regulators, and public servants offer the institutional knowledge needed to craft laws that are not only forward-looking but also grounded in practical enforceability. Their expertise ensures that AI governance is realistic, ethical, and just (OECD.AI, 2021).
Finally, in digital literacy, retired educators and technically proficient seniors are bridging generational divides. Through community workshops, mentoring programs, and partnerships with organizations like AARP and TechSoup, they help demystify AI for older adults—empowering their peers to engage with emerging technologies rather than fear them (Dono, 2021; TechSoup, 2016).
Whether it’s shaping green tech, guiding regulation, or bridging the digital divide, retirees continue to play a powerful role in making AI more inclusive, usable, ethical and sustainable.
Implementation Frameworks: Turning Insight into Integration
For organizations seeking to tap into WisGen expertise, having structured models can make engagement both effective and repeatable. A WisGen Inclusion Framework could include:
- Partnering with alumni associations, senior citizen professional networks, or retirement-focused organizations (e.g., AARP, SeniorNet, Encore.org).
- Creating interdisciplinary advisory boards that include both tech experts and retired professionals with domain-specific experience.
- Offering flexible roles such as part-time fellowships, consulting arrangements, or volunteer opportunities that recognize the diverse motivations of retirees.
- Normalizing intergenerational collaboration within AI teams and institutions through formal integration processes.
On the other hand, while many retirees are already digitally proficient, it’s important to recognize that others may need support navigating modern AI tools and platforms. Upskilling initiatives—such as AI-literacy bootcamps, interactive workshops, or peer-led learning groups—can empower more WisGen members to contribute meaningfully. Organizations can also accelerate inclusion by developing onboarding materials tailored to non-technical professionals, helping bridge the initial familiarity gap with emerging AI systems and terminology.
To reinforce the case for investing in WisGen participation, organizations should also prioritize data and measurement. Empirical evidence strengthens the case for policy and practice. Key metrics to track might include:
- Bias reduction outcomes before and after WisGen engagement in design or dataset reviews.
- Improvements in explainability and user trust, as measured through usability testing or stakeholder feedback.
- ROI metrics for mentorship programs, such as time-to-productivity for junior developers, decreased error rates, or improved deployment outcomes.
Publishing these findings can not only build internal momentum but also encourage broader adoption of WisGen collaboration across industries.
Conclusion
AI is only as ethical, fair, and effective as the humans guiding it. And in this era of rapid advancement, what the field needs most isn’t more innovation—it’s more wisdom.
WisGen offers that wisdom in abundance. With decades of experience navigating complex systems, making high-stakes decisions, and upholding ethical standards, they are uniquely positioned to help shape AI that works not just in theory, but in the messy realities of the world. Whether it’s correcting bias, improving explainability, protecting privacy, stress-testing systems, or mentoring the next generation, their contributions are not merely beneficial—they are essential.
Their insights ground AI in lived human experience. They remind us that technology should serve people—not the other way around. If AI is to truly be responsible, inclusive, and sustainable, we must invite those who’ve spent their lives solving hard problems to the table—to help build what comes next.
References
- AI Multiple. (2025, March 22). AI bias: Definition, types & mitigation strategies. https://research.aimultiple.com/ai-bias/
- Bain, M. (2024, March 1). Legal transparency in AI finance: Facing the accountability dilemma in digital decision-making. Reuters. https://www.reuters.com/legal/transactional/legal-transparency-ai-finance-facing-accountability-dilemma-digital-decision-2024-03-01/
- Bender, E. M., Gebru, T., McMillan-Major, A., & Shmitchell, S. (2021). On the Dangers of Stochastic Parrots: Can Language Models Be Too Big? Proceedings of the 2021 ACM Conference on Fairness, Accountability, and Transparency (FAccT ’21), 610–623. https://doi.org/10.1145/3442188.3445922
- Dono, L. (2021, February 8). AARP joins with nonprofit to teach tech to older adults. AARP. https://www.aarp.org/about-aarp/info-2021/oats-senior-planet.html
- Early, C. (2024, November 28). Sustainability profession scrambles to fill ‘extreme gap’ in digital skills to harness power of AI. Reuters. https://www.reuters.com/sustainability/society-equity/sustainability-profession-scrambles-fill-extreme-gap-digital-skills-harness-2024-11-28/bizwireexpress.com+3Reuters+3CSRwire+3
- Evolving AI. (2023, October 12). McKinsey developed an internal AI tool called Lilli, trained on over 100,000 internal documents and interviews spanning a century of the firm’s knowledge. LinkedIn. https://www.linkedin.com/posts/evolving-ai_mckinsey-developed-an-internal-ai-tool-called-activity-7323698907195052032-oLUK/?utm_source=share&utm_medium=member_ios&rcm=ACoAAACForcB-Kg0fhmDtzuGUcK3nOlpRHM0038
- Hinchliffe, E. (2023, July 21). AI regulation pushes for explainability. TIME. https://time.com/6289953/schumer-ai-regulation-explainability/
- IEEE. (2019). Ethically aligned design: A vision for prioritizing human well-being with autonomous and intelligent systems (1st ed.). https://ethicsinaction.ieee.org/
- Mariwala, V., & Sundharam, J. (2025, May 13). AI has a bias against the elderly. It’s bad for business. The Print. https://theprint.in/opinion/ai-has-a-bias-against-the-elderly-its-bad-for-business/2622046/
- Marr, B. (2018, June 25). The AI skills crisis and how to close the gap. Forbes. https://www.forbes.com/sites/bernardmarr/2018/06/25/the-ai-skills-crisis-and-how-to-close-the-gap/
- MentorCruise. (n.d.). Home. Retrieved May 5, 2025, from https://mentorcruise.com/
- MIT Venture Mentoring Service. (n.d.). Home. Massachusetts Institute of Technology. Retrieved May 5, 2025, from https://vms.mit.edu
- OECD.AI. (2021). OECD.AI Dashboard Overview. Organisation for Economic Co-operation and Development. Retrieved May 5, 2025, from https://oecd.ai/en/dashboards/overview
- Singla, A., Sukharevsky, A., Yee, L., Chui, M., & Hall, B. (2024, May 30). The state of AI in early 2024: Gen AI adoption spikes and starts to generate value. McKinsey & Company. https://www.mckinsey.com/capabilities/quantumblack/our-insights/the-state-of-ai-2024
- Stanford Institute for Human-Centered Artificial Intelligence. (2024, August 6). Testing AI in health care requires human judgment. https://hai.stanford.edu/news/testing-ai-health-care-requires-human-judgment
- Stack, L. (2023, May 27). Here’s what happens when your lawyer uses ChatGPT. The New York Times. https://www.nytimes.com/2023/05/27/nyregion/avianca-airline-lawsuit-chatgpt.html
- TechSoup. (2016, May 18). Digital skills for older adults: Teaching technology in public libraries [Webinar transcript]. https://www.techsoup.org/sitecollectiondocuments/webinar-digital-skills-for-older-adults-teaching-technology-2016-05-18-transcript.pdf
- Times of India. (2024, April 5). Experts highlight ethical concerns in AI: Algorithmic bias, data privacy, and user autonomy. https://timesofindia.indiatimes.com/india/experts-hightlight-ethical-concerns-in-ai-algorithmic-bias-data-privacy-and-user-autonomy/articleshow/118889538.cms
- Toulas, B. (2024, March 12). Over 12 million auth secrets and keys leaked on GitHub in 2023. BleepingComputer. https://www.bleepingcomputer.com/news/security/over-12-million-auth-secrets-and-keys-leaked-on-github-in-2023/
- Van Zyl, J. (2024, January 25). How the demands of AI are impacting data centers and what operators can do. TechHQ. https://techhq.com/2024/01/how-the-demands-of-ai-are-impacting-data-centers-and-what-operators-can-do/
- Vincent, J. (2023, May 27). ChatGPT cited non-existent cases. Why didn’t the lawyers check? The Verge. https://www.theverge.com/2023/5/27/23739913/chatgpt-ai-lawsuit-avianca-airlines-chatbot-research
- WEF. (2023). AI governance requires diverse voices. https://www.weforum.org/agenda/2023/07/ai-governance-diverse-voices-inclusive/